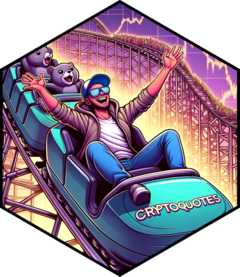
Converting XTS and ZOO objects
What about data integrity?
Source:vignettes/articles/04-converting.Rmd
04-converting.Rmd
## load library
library(cryptoQuotes)
If you want to use the simplicity of tidverse
or the
power of data.table
, the xts
-object can be
easily converted.
However, its important to maintain data integrity, especially, if the date and timezone is important for you.
Converting xts and zoo to tibble
Converting to tibble
requires a few steps to achieve the
same data structure as the xts
,
# 1) load pipe
library(magrittr)
# 2) convert to tibble
# using as_tibble
tbl <- tibble::as_tibble(
x = cbind(
Index = zoo::index(ATOM),
zoo::coredata(ATOM)
)
) %>% dplyr::mutate(
Index = lubridate::as_datetime(
Index
)
)
# 3) head data
head(tbl, 3)
#> # A tibble: 3 × 6
#> Index open high low close volume
#> <dttm> <dbl> <dbl> <dbl> <dbl> <dbl>
#> 1 2023-12-29 23:00:00 10.9 10.9 10.9 10.9 3260.
#> 2 2023-12-29 23:15:00 10.9 11.0 10.9 11.0 1863.
#> 3 2023-12-29 23:30:00 11.0 11.0 10.9 11.0 1861.
Converting xts and zoo to data.table
Converting to data.table
is straightforward as
as.data.table()
handles everything under the hood,
# 1) convert to data.table
# using as.data.table
DT <- data.table::as.data.table(
ATOM
)
# 2) set column name to upper
colnames(DT)[1] <- 'Index'
# 3) head data
head(DT, 3)
#> Index open high low close volume
#> <POSc> <num> <num> <num> <num> <num>
#> 1: 2023-12-30 00:00:00 10.8778 10.9133 10.8775 10.9089 3259.760
#> 2: 2023-12-30 00:15:00 10.9089 10.9530 10.9008 10.9512 1862.697
#> 3: 2023-12-30 00:30:00 10.9512 10.9884 10.9373 10.9832 1861.493
Checking data integrity
Checking date integrity
It is important that the date.time has not been converted to a different timezone in the process, without explicitly coding it as such,
Checking OHLCV values
It goes without saying that R
-functions wouldn’t tamper
with the order of the data during conversion without a warning in the
documentation, but nonetheless for the sake of argument, we will check
the OHLCV values,
# 1) store price objects
# Open price here
open_price <- list(
tbl = tbl$Open,
DT = DT$Open
)
#> Warning: Unknown or uninitialised column: `Open`.